
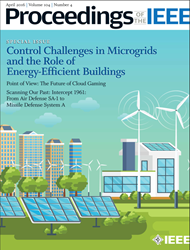

" An Overview of the Building Energy Management System Considering the Demand Response Programs, Smart Strategies and Smart Grid,"Įnergies, MDPI, Open Access Journal, vol. Rokonuzzaman & Foo Wah Low & Chong Tak Yaw & Nilofar Asim & Nurul Asma Samsudin & Sieh Kiong Tiong & Chong Kok Hen & Chin Wei Lai, 2020.

Mohammad Shakeri & Jagadeesh Pasupuleti & Nowshad Amin & Md." Fuzzy compromised solution-based novel home appliances scheduling and demand response with optimal dispatch of distributed energy resources," Waseem, Muhammad & Lin, Zhenzhi & Liu, Shengyuan & Zhang, Zhi & Aziz, Tarique & Khan, Danish, 2021." Analysis and optimization of HVAC control systems based on energy and performance considerations for smart buildings," repec:gam:jeners:v:8:y:2015:i:11:p:13112-13136:d:59014 is not listed on IDEASįull references (including those not matched with items on IDEAS)." The evolution of electricity demand and the role for demand side participation, in buildings and transport,"Įnergy Policy, Elsevier, vol. Barton, John & Huang, Sikai & Infield, David & Leach, Matthew & Ogunkunle, Damiete & Torriti, Jacopo & Thomson, Murray, 2013." A Novel Quantum-Behaved Lightning Search Algorithm Approach to Improve the Fuzzy Logic Speed Controller for an Induction Motor Drive,"Įnergies, MDPI, Open Access Journal, vol. Jamal Abd Ali & Mahammad A Hannan & Azah Mohamed, 2015." A novel PSO (Particle Swarm Optimization)-based approach for optimal schedule of refrigerators using experimental models,"Įnergy, Elsevier, vol. Farzamkia, Saleh & Ranjbar, Hossein & Hatami, Alireza & Iman-Eini, Hossein, 2016." A Nature-Inspired Optimization-Based Optimum Fuzzy Logic Photovoltaic Inverter Controller Utilizing an eZdsp F28335 Board,"Įnergies, MDPI, Open Access Journal, vol. Ammar Hussein Mutlag & Azah Mohamed & Hussain Shareef, 2016.

The proposed scheduling algorithm can significantly reduce the peak-hour energy consumption during the DR event by up to 9.7138% considering four appliances per 7-h period. Results show that the hybrid LSA-ANN outperforms the hybrid PSO based ANN. Results of the hybrid LSA-ANN are compared with those of hybrid particle swarm optimization (PSO) based ANN to validate the developed algorithm. Therefore, the ON/OFF estimation accuracy by ANN can be improved. Given that finding proper ANN tuning parameters is difficult, the LSA optimization is hybridized with ANN to improve the ANN performances by selecting the optimum values of neurons in each hidden layer and learning rate. The ANN controller has to be tuned properly using suitable learning rate value and number of nodes in the hidden layers to schedule the appliances optimally. Four appliances, namely, air conditioner, water heater, refrigerator, and washing machine (WM), are developed by Matlab/Simulink according to customer preferences and priority of appliances. In the proposed approach, a set of the most common residential appliances are modeled, and their activation is controlled by the hybrid LSA-ANN based home energy management scheduling controller. Consequently, the scheduled operation of several appliances is improved in terms of cost savings. In this study, the home energy management scheduling controller of the residential DR strategy is proposed using the hybrid lightning search algorithm (LSA)-based artificial neural network (ANN) to predict the optimal ON/OFF status for home appliances. Demand response (DR) program can shift peak time load to off-peak time, thereby reducing greenhouse gas emissions and allowing energy conservation.
